AI in Risk Management: Applications, Challenges, and Best Practices
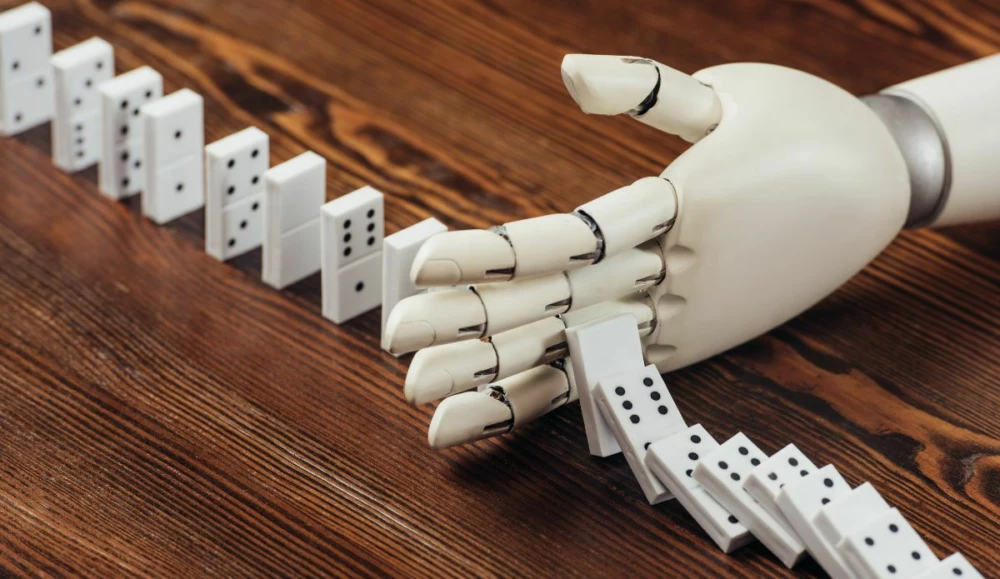
Artificial intelligence (AI) has a unique relationship to risk. AI capability is popping up in virtually every business softwarel, and it’s happening at a rate (and with a degree of uncertainty) that amplifies the potential for risk. On the other hand, the processing power of AI makes it a powerful tool for managing risks—including third-party risks. It can seem like a bit of a “snake eating its own tail” situation.
AI risk management is the process of untangling AI's risk from its utility—and there's enormous value in getting it right. Let’s take a look at the challenge from every angle: how we define AI risk management, how it can be used to reduce risk, risks inherent to the AI itself, and how to create a risk-management framework for your own AI tools.
What Is AI Risk Management?
Let’s start with the basics:
AI risk management involves the identification, assessment, and mitigation of risks associated with AI technologies. It focuses on ensuring AI systems operate securely, ethically, and in alignment with an organization’s goals and compliance requirements.
As we mentioned before, it’s also useful to expand this essential definition to include risk-management approaches that leverage AI systems to accomplish goals. We’ll be discussing these in a moment.
Unlike traditional software, AI systems often involve machine learning (ML), deep learning, or generative AI, which rely on large datasets and algorithms to make decisions. This creates unique risks, such as model bias, explainability challenges, and heightened security vulnerabilities, which traditional risk management approaches cannot fully address.
For example, AI systems used in fraud detection must accurately analyze financial transactions without introducing unintended biases or producing excessive false positives. This is a use case in which an AI solution is used as part of the risk-management process, but is also itself a potential source of risk. Effective AI risk management ensures such systems are reliable, transparent, and secure.
Now, let’s take a look at some of the ways AI can enhance your risk-management practices.
Applications of AI in Risk Management
AI allows businesses to analyze vast amounts of data, automate routine processes, and predict potential threats. While human oversight and judgment is a huge part of excellent risk management, the benefits of AI may allow you to identify risks more easily, utilize data sources in your assessments that may not have been readily available in the past, and detect anomalies that the human eye might miss. Below are some of the key applications of AI in various risk management domains:
1. Fraud detection and prevention
AI-powered systems are highly effective in identifying fraudulent activities in real-time. By analyzing patterns in transaction data, AI can flag anomalies that may indicate fraud, such as unusual spending behaviors or access attempts. Machine learning algorithms continuously improve their detection capabilities, reducing false positives and enhancing accuracy.
2. Cybersecurity
AI plays a pivotal role in strengthening cybersecurity defenses. It can:
- Detect and respond to suspicious behavior on networks
- Analyze security logs to identify vulnerabilities
- Prevent phishing attacks by detecting fraudulent emails
- Quickly creating resources and guidelines to enhance cybersecurity awareness and training
AI-driven cybersecurity solutions provide organizations with proactive tools to combat evolving cyber threats, but it should be viewed as simply another arrow in the quiver when it comes to defending against cyber threats. Great cybersecurity still relies on a multi-dimensional approach that includes governance, threat identification, preventative measures, threat mitigation and response, and recovery. AI can be an enhancement to many of these steps.
3. Compliance and regulatory risk
AI simplifies compliance by automating data collection, reporting, and monitoring activities. For instance, natural language processing (NLP) tools can analyze legal documents to ensure policies align with regulatory requirements. AI systems can also flag non-compliance risks before they escalate.
4. Operational risk management
Operational risks, such as supply chain disruptions or system failures, can be mitigated using AI. Predictive analytics enables businesses to foresee potential bottlenecks or failures and implement corrective actions. For example, AI can optimize supply chain logistics by forecasting demand and ensuring efficient resource allocation.
5. Credit risk assessment
AI improves the accuracy of credit risk evaluations by analyzing a broad range of customer data, including payment histories and financial behaviors. This enables lenders to make more informed decisions while reducing the likelihood of default.
AI in Third-Party Risk Management
As a subset of overall risk-management, third-party risk management (TPRM; sometimes called vendor risk management, or VRM), is especially critical. That’s because organizations are increasingly reliant on vendors for essential business functions—Whistic data shows a 12% year-over-year increase in the number of organizations working with 100 or more vendors. Data also shows that 88% of recent breaches (those happening within the last three years) originated with a third party.
These trends suggest that TPRM needs to be a core pillar of overall risk management, and there is a huge opportunity to leverage AI to make this process more effective and deliver greater business value. Here’s how AI can help you build a stronger TPRM program.
AI-driven TPRM creates opportunities for automation and greater insight
Third-party risk management is the process of evaluating the inherent risks of a vendor before they have access to important systems and data. This evaluation, called a vendor security assessment, usually takes the form of a questionnaire to collect information relevant to your security or regulatory requirements.
These questionnaires can be based on established, industry-standard frameworks like ISO or NIST, and they can also be customized to reflect the unique needs of your business. A completed questionnaire gives your business all the information you need to demonstrate due-diligence, evaluate and prove regulatory compliance, and evaluate the risk vs. reward of a given vendor based on your organization’s overall risk appetite.
For many companies, though, there are major challenges and headaches associated with the TPRM process. The traditional approach to TPRM is highly manual and time-consuming. In response, resource-strapped risk teams must either take weeks or months to complete an assessment—or simply take on more risk and hope for the best.
AI allows organizations to tackle these challenges by automating and improving the process for both buyers and vendors by:
- Utilizing a wider range of security data. Rather than relying strictly on the choke point of the assessment questionnaire (which can be arduous for both sides), AI allows you to access information from a variety of sources, like Trust Centers, completed audit reports like SOC 2s, or previously completed questionnaires.
- Sourcing answers to even customized questionnaires. AI can be trained on your security posture, and when given access to a repository of security data, can identify specific answers to your questions. This can also be used by vendors to automatically complete incoming requests. AI language models can also understand the semantic intent of a question, so this even works for customized questionnaires.
- Maintaining transparency and control. AI in platforms like Whistic only access the information or documentation you approve. This means you can create a pre-approved repository of documentation for assessments—greatly reducing the risk of proprietary information leaking. When it comes to transparency, AI-driven TPRM provides a rationale for AI-generated answers, along with both a confidence score and citations for its responses.
Challenges in AI Risk Management
Of course, in both overall risk management and TPRM, the use of AI can introduce some challenges. Let’s take a look at what those challenges are and how to address them.
1. Data quality and bias
AI systems rely on large datasets for training. If these datasets contain biases or inaccuracies, the resulting models may produce flawed decisions. For example, biased training data can lead to discriminatory lending practices or inaccurate fraud detection.
2. Lack of explainability
Many AI models operate as "black boxes," meaning their decision-making processes are not easily interpretable. This lack of transparency poses challenges for regulatory compliance and stakeholder trust, particularly in high-stakes industries such as healthcare and finance.
3. Security vulnerabilities
AI systems can themselves become targets for cyberattacks. Adversarial attacks, in which malicious actors manipulate AI models, can lead to inaccurate predictions or compromised security outcomes.
4. Regulatory complexity
The rapid evolution of AI has outpaced regulatory frameworks, creating uncertainty around compliance requirements. Businesses must navigate diverse regulations, such as GDPR in Europe or the AI Risk Management Framework by NIST, to ensure their AI systems meet legal standards. More on these in a moment.
5. Integration and scalability
Integrating AI systems into existing workflows can be challenging, particularly for organizations with limited technical expertise. Poor integration may result in inefficiencies or security gaps.
Understanding these challenges is an important step in terms of both mitigating risk and for leveraging the value of AI to improve risk-management outcomes. But it’s not all doom and gloom; there are some common steps that every organization can take to avoid these pitfalls and get the most out of AI risk management.
Best Practices for Implementing AI Risk Management
To effectively manage the risks associated with AI technologies, businesses should adopt a strategic approach that prioritizes transparency, security, and alignment with organizational goals. To achieve these goals, it’s important to:
1. Develop clear AI governance policies
Establishing governance frameworks ensures accountability and consistency in AI use. Governance policies should address:
- The purpose of AI deployment and how AI is utilized in the solution or as part of its development
- Ethical considerations, such as bias mitigation
- Roles and responsibilities for AI oversight. By documenting guidelines and collaborating regularly, organizations create a foundation for responsible AI use.
2. Invest in high-quality, diverse data
To minimize the risk of biased or inaccurate AI models, businesses must prioritize data quality. This includes:
- Regularly auditing training datasets for biases.
- Incorporating diverse data sources to improve model generalization.
- Implementing data validation protocols to ensure accuracy. High-quality data strengthens the reliability of AI outputs and builds stakeholder trust.
3. Utilize Explainable AI (XAI)
Explainable AI tools provide insights into how AI models make decisions. By enhancing transparency, XAI enables businesses to:
- Justify AI-driven decisions to regulators and stakeholders
- Identify and address potential flaws in model behavior
- Build confidence in AI systems among end-users
4. Incorporate AI-specific risk frameworks
Standard frameworks improve risk-management outcomes by addressing a wide variety of risk types and making recommendations. Similar frameworks are beginning to emerge to help evaluate AI risk. These frameworks include:
- NIST AI Risk Management Framework—The National Institute of Standards and Technology (NIST) is a trusted authority on technology frameworks. Like their previous frameworks around cybersecurity and data privacy, NIST’s AI framework focuses on AI risk characterization; recommendations for data management and governance; transparency in AI development and deployment; explainability and interpretability; and human collaboration with AI systems.
- capAI, based on the European Union’s AI Act—The AI Act, adopted by the European Parliament on June 14, 2023, is designed to ensure that AI technology is secure, transparent, traceable, unbiased, and environmentally sound. The act designates risk levels for AI systems, with special provisions for the use of generative AI.
- ISO 23053—This framework was created by the International Organization for Standardization (ISO) in June 2022 and is specifically designed to assess AI and Machine Learning systems. ISO 23053 establishes a common terminology and core concepts for these systems, describes AI components and functions, and applies to public and private organizations of all sizes. Whistic has created a security assessment questionnaire based on ISO 23053.
5. Conduct regular security assessments
AI systems should undergo regular security assessments to identify vulnerabilities. This includes:
- Penetration testing to evaluate system defense.
- Monitoring for adversarial attacks
- Implementing robust encryption protocols to protect data. Security assessments are crucial for maintaining the integrity of AI systems in dynamic threat landscapes.
- Regular vendor assessments as AI adoption proliferates across supply chains
6. Foster cross-department collaboration
Effective AI risk management requires input from multiple stakeholders, including IT, InfoSec, Legal, and Compliance teams. Collaboration ensures that AI systems align with business goals and regulatory requirements. For example:
- IT and InfoSec teams can address technical risks, such as integration challenges.
- Legal teams can ensure compliance with evolving regulations.
- Compliance teams can monitor adherence to ethical standards.
7. Automate risk monitoring
AI-powered monitoring tools can enhance risk management by automating the detection of potential issues. For instance:
- Continuous monitoring tools can identify changes in vendor risk profiles.
- Automated alerts can notify teams of policy violations or unusual activity. Automation reduces the burden on risk management teams while improving efficiency.
The Future of AI Risk Management
As AI continues to evolve, its role in risk management will expand. Emerging technologies, such as generative AI and deep learning, offer new capabilities for identifying and mitigating risks. However, businesses must remain vigilant in addressing the challenges these technologies introduce.
Future trends in AI risk management include:
- Integration with cybersecurity: AI tools will play a larger role in detecting and preventing cyber threats, such as ransomware attacks or data breaches.
- Regulatory advancements: Governments and industry organizations will develop more robust regulations to govern AI use, creating greater clarity for businesses.
- Increased emphasis on ethics: Businesses will prioritize ethical AI practices, such as bias mitigation and responsible data use, to build trust with customers and stakeholders.
- Modern, AI-first TPRM: AI will make it possible to assess more vendors and respond to more requests in a fraction of the time; it will also make it possible to conduct more robust assessments and empower greater insight.
Whistic is the modern approach to AI third-party risk management
AI is a transformative force in risk management, enabling businesses to detect threats, enhance efficiency, and maintain compliance. But its adoption requires careful consideration of unique risks, such as data quality, explainability, and security vulnerabilities.
By implementing best practices—such as investing in high-quality data, utilizing explainable AI, and incorporating established risk frameworks—organizations can harness the power of AI while mitigating its risks. As AI technologies continue to advance, proactive risk management will be essential for ensuring their safe and effective use.
In fact, that future is already here. The Whistic Platform utilizes a powerful AI engine to automate the vendor assessment on both sides. Vendors can automate the response process and use Whistic’s Trust Catalog information exchange to even deflect incoming questionnaires, while buyers can automate responses and source detailed answers to their questions with Whistic’s Assessment Copilot:
- Summarize complex security documentation with SOC 2 Summaries
- Source the widest range of vendor data sources to complete assessments with Vendor Summary
- Query your entire vendor catalog for global security answers with Vendor Insights
Whistic customers also have access to a growing library of more than 50+ standards and frameworks, including NIST. That means your ability to assess AI risk also improves in the platform.
Effective, secure AI risk management is possible. Schedule a demo today to learn how Whistic can support your program.