How to Think About AI Risk: Key Questions and Frameworks
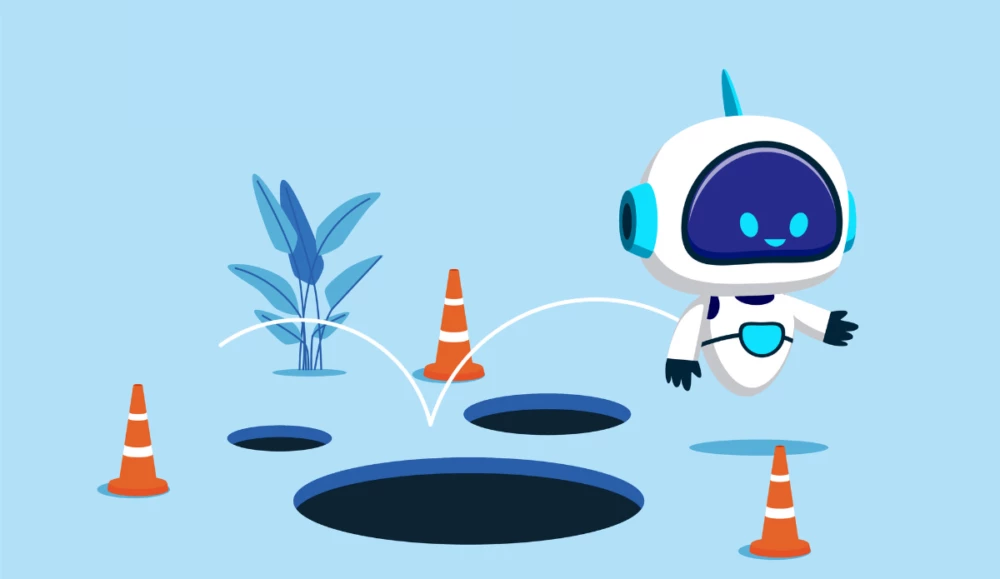
With the proliferation of artificial intelligence across the vendor and third-party landscape, the ability to identify and manage AI risks is increasingly important. It’s essential that your third-party risk management (TPRM) practices include a systemized way to assess the AI risk of your vendor inventory.
Let’s take a look at the elements that make up a risk-based approach to AI vendor security. We’ll examine the core questions your program must answer; look at some of the key challenges; and review the latest NIST framework and its recommendations for assessing AI risk.
Assessing AI Risks: Essential Questions
Building AI risk into your TPRM program can be challenging given the pace at which AI is evolving. To begin, it’s important to answer the following questions about the use of AI in your third-party ecosystem:
- Which third parties are using AI, and what are they using it for? Your vendors may be using AI capabilities in their products, as part of their development process, or both. Understanding how AI is used in your vendor products helps to establish the scope of things you need to assess for and determine which stakeholders to engage in the process.
- How do your vendors approach AI security controls during the development phase? Once you understand how the vendors are utilizing AI, make sure they are able to document and share the controls they used as they were building their products and AI models.
- How is my data used by the AI models? Be able to document how, precisely, your data is used by AI. For example, some vendors may use customer data to train AI models to improve their products and services. Depending on the kind of data your vendor has access to, this may represent a greater risk.
- What controls do I have? To minimize risk, you should be able to control which data is accessed, how it’s used by the AI models, and when it’s used. This allows you to maintain visibility and opt in or out of using AI capabilities.
Challenges of Assessing AI in TPRM
The ability to answer these questions with confidence is essential because there are still barriers to clear before AI security assessments are mature and can keep pace with the rapid evolution of the technology. Here are some of the core challenges to keep in mind as you build and implement your risk-based approach to AI:
- Are you able to measure AI risk? As of now, there are not reliable, consistent, battle-tested metrics for quantifying AI risk or trustworthiness. This may improve as use cases accumulate, but there may also be a disconnect between the metrics a developer or vendor uses to measure AI risk and your own methodologies.
- What level of risk is acceptable? It may be difficult to clearly articulate your risk tolerance for AI early in your adoption. Risk tolerance is always relative and contextual, but this is especially the case with AI, where competitive advantages are not always proven. This makes it more challenging to weigh risk vs. reward as you might with other, more established technologies.
- How do I prioritize AI risk? Without industry-standard risk metrics or clearly defined risk tolerance, it can be difficult to know how many and what kinds of resources to dedicate to AI risk monitoring and remediation. Allocate too few resources, and you expose yourself to additional risks; but too resources may impact efficiency and undercut the business value of AI.
- How does AI risk management fit into my broader risk strategy? Risk management can’t be ad hoc; it requires an organization-wide discipline—and this will eventually require a cogent, integrated approach to AI risk.
- Who are the key stakeholders to manage AI risk? This relates closely to your overall risk strategy, but you may need to include additional parties in decision-making around AI procurement, ongoing risk monitoring of AI solutions, and incident response that differ from your traditional program.
Incorporating Frameworks into AI Risk Management
It may seem that there are more questions than answers when it comes to assessing AI risk in your vendors or solutions. When compared to more established technologies—whose risks are well-understood and documented—there is some truth to this notion.
But while there are still unknowns around AI risk, your overall VRM approach can still leverage many existing best practices. These can come from your own experiences, or they can draw from standardized frameworks. These are still in the nascent stages, but the National Institute of Standards and Technology (NIST) has developed an AI Risk Management Framework (AI RMF) that can be a helpful starting point for building and benchmarking your program.
Here are the core pillars of the AI RMF:
1. Governance of AI Risk
This step in the process is designed to codify processes, practices, and stakeholders that form the foundations of your approach to AI risk. NIST recommends breaking down Governance into tiers of activity:
- Develop policies and procedures—This helps to establish an organization-wide culture of AI risk by creating consistent guidelines for mapping and measuring. If you don’t currently have a clear picture of your risk tolerance related to AI, this will be a starting point for metrics.
- Define roles and responsibilities—Create clear expectations for stakeholder engagement, create systems of accountability to ensure cooperation and adherence to process, and identify opportunities for ongoing training and skill development.
- Codify, clarify, and refine approach over time—Ensure your governance plan includes mechanisms for continuous improvement. Your stakeholders should meet on a regular basis, develop systems of reporting based on shared metrics, and be prepared to revise standards as AI risk management evolves.
2. Mapping AI Across the Organization
This stage of the framework is intended to increase overall visibility into the ways AI systems are interacting across the complex environment of a large organization. Decisions made in one part of the business can actually change AI behaviors. As NIST notes, “The interdependencies between [AI-related] activities, and among relevant AI actors, can make it difficult to reliably anticipate impacts of AI systems.”
Mapping improves the capacity of an organization to understand this AI context and makes it easier to check assumptions against actual behaviors. At this stage in the process, NIST recommends documenting:
- Expected outcomes from the AI system
- Business value of the solution or capability
- Defined risk tolerance (even if it’s just to establish an initial baseline)
- Defined limits of AI knowledge acquisition and rules for control
- Scope of AI use across your environment
3. Measurement and Reporting
In this portion of the framework, NIST recommends establishing initial baselines for consistent measurement of performance, trustworthiness, and overall risk of AI. As we mentioned earlier, such clear metrics may be in nascent stages for your business. But NIST recommends a few activities to focus on to build a robust measurement engine:
- Utilize your mapping to identify highest-risk areas and focus there first
- Identify trusted third party auditors to assess your AI usage and validate your approach
- Develop recurring testing of your metrics
- Keep ongoing documentation about AI behaviors
- Perform assessments for a variety of risk types (i.e. resilience, privacy, bias, sustainability) to your AI systems
Whistic’s AI-First TPRM Platform is Built on Trust and Transparency
Assessing third-party risks of all kinds can be an enormous challenge—and AI makes that challenge even more complex. That’s why our TPRM platform leverages AI capabilities to automate the assessment process and provide context-rich data for your decision making.
But we also understand the risks that come with evolving technologies like AI, which is why we lead with trust and transparency. Whistic AI is:
- Fully transparent. We offer our customers full access to our trust center, so you always understand how we use AI and what it means for our solutions.
- Built on trust. Whistic does not use your proprietary data to train our model. We maintain robust security and privacy controls to protect your information while ensuring confidentiality, regulatory compliance, and resilience against cyber threats.
- Customer lead. You maintain control of Whistic AI. You can turn it off or on at any time, and you can always fully audit AI-generated responses to security questions for accuracy.
- Context-rich. For every automated assessment with Whistic’s AI-powered Assessment Copilot, each response will include a confidence and relevance score, full document citations, and links to relevant places in those documents so you can do a deeper dive.
We want to make it easier for you to assess all the vendors you need in a fraction of the time, so you can better manage risks. And we want to make it easier for you to trust and utilize AI in this process. Let our team of experts show you how Whistic AI works for businesses like yours by booking a quick demo today.